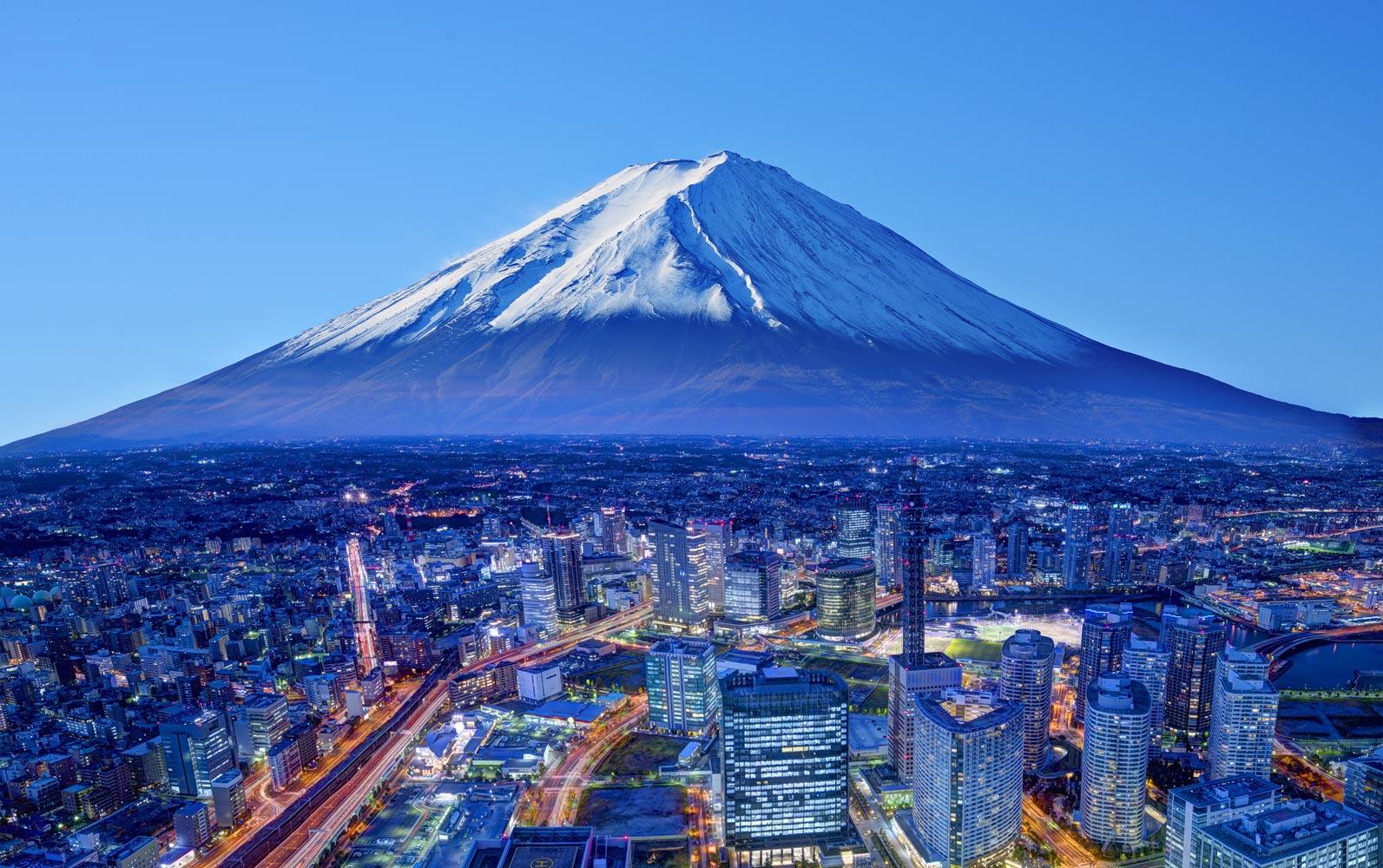
Q-learning
The Q-learning hindrance avoidance algorithm.
The Q-learning obstacle avoidance algorithm based upon EKF-SLAM for NAO autonomous walking less than unidentified conditions
The 2 important problems of SLAM and Pathway organizing are usually tackled alone. However, both are essential to achieve successfully autonomous navigation. With this document, we try to incorporate both qualities for app with a humanoid robot. The SLAM problem is sorted out using the EKF-SLAM algorithm while the path preparation problem is tackled by means of -studying. The recommended algorithm is carried out with a NAO provided with a laser light go. In order to distinguish different landmarks at a single viewing, we utilized clustering algorithm on laser light sensing unit details. A Fractional Buy PI controller (FOPI) is likewise created to reduce the movements deviation inherent in in the course of NAO’s wandering behavior. The algorithm is tested within an indoor atmosphere to gauge its efficiency. We propose the new style can be reliably useful for autonomous wandering inside an unknown environment.
The 2 important problems of SLAM and Pathway organizing are usually tackled alone. However, both are essential to achieve successfully autonomous navigation. With this document, we try to incorporate both qualities for app with a humanoid robot. The SLAM problem is sorted out using the EKF-SLAM algorithm while the path preparation problem is tackled by means of -studying. The recommended algorithm is carried out with a NAO provided with a laser light go. In order to distinguish different landmarks at a single viewing, we utilized clustering algorithm on laser light sensing unit details. A Fractional Buy PI controller (FOPI) is likewise created to reduce the movements deviation inherent in in the course of NAO’s wandering behavior. The algorithm is tested within an indoor atmosphere to gauge its efficiency. We propose the new style can be reliably useful for autonomous wandering inside an unknown environment.
Strong estimation of jogging robots tilt and velocity using proprioceptive detectors info fusion
A technique of tilt and velocity estimation in mobile, perhaps legged robots according to on-board detectors.
•Robustness to inertial sensor biases, and findings of inferior or temporal unavailability.
•A straightforward platform for modeling of legged robot kinematics with foot angle taken into account.
Accessibility of the immediate speed of any legged robot is normally necessary for its productive management. However, estimation of velocity only on the basis of robot kinematics has a significant drawback: the robot is not in touch with the ground all the time, or its feet may twist. In this paper we present a method for velocity and tilt estimation in the jogging robot. This procedure mixes a kinematic kind of the supporting lower leg and readouts from an inertial sensor. You can use it in virtually any ground, regardless of the robot’s body style or maybe the management strategy employed, in fact it is strong when it comes to ft . angle. It is also safe from minimal foot push and temporary insufficient foot contact.
For more information about Q-learning net page: look at here.
A technique of tilt and velocity estimation in mobile, perhaps legged robots according to on-board detectors.
•Robustness to inertial sensor biases, and findings of inferior or temporal unavailability.
•A straightforward platform for modeling of legged robot kinematics with foot angle taken into account.
Accessibility of the immediate speed of any legged robot is normally necessary for its productive management. However, estimation of velocity only on the basis of robot kinematics has a significant drawback: the robot is not in touch with the ground all the time, or its feet may twist. In this paper we present a method for velocity and tilt estimation in the jogging robot. This procedure mixes a kinematic kind of the supporting lower leg and readouts from an inertial sensor. You can use it in virtually any ground, regardless of the robot’s body style or maybe the management strategy employed, in fact it is strong when it comes to ft . angle. It is also safe from minimal foot push and temporary insufficient foot contact.
For more information about Q-learning net page: look at here.